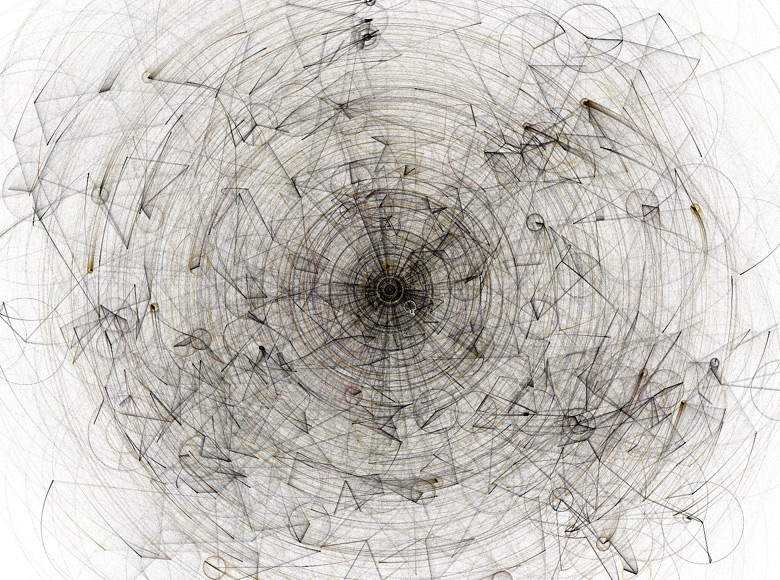
Most would agree that it has been a year that feels like it was drawn from the storyline of a particularly lurid B-movie. Even by those standards, though, the comment “I am afraid your grades were almost derailed by a mutant algorithm” was one that stood out, painting a picture of Britain’s youth falling victim to some out of control, unaccountable, technological mystery.
Algorithms of course will not be mysterious to the regular readers of this newsletter. They are an increasingly ubiquitous part of the discussion around technology and the law (see eg my article in the March 2019 issue). But in recent years, the concept of algorithms being to blame for the harms done by technology has started to catch on in the wider public consciousness. And in accordance with the old legal maxim ubi culpa est, ibi est petition (where there’s blame, there’s a claim), the question “what is an algorithm anyway?” is rapidly being overtaken by “so who can I sue for the harm this rogue computer software has done?”
Who is accountable?
This is, in fact, a surprisingly good question. Until pretty recently, accountability for the workings of computer software was a relatively simple matter. Software either came packaged in a box from a known distributor, or was coded on a bespoke basis by a developer or team, according to a particular client’s specifications. In each case the product would be warranted to a certain (fairly basic) standard of fitness. The buyer – who would have deployed the software on their own system – would look to the vendor in the event that the software did not perform as advertised.
The more sophisticated computer software has become, however, the more challenging the attribution of responsibility. It can be tempting, particularly for those who have been tasked with delivering a particular outcome through technology, simply to blame the “mutant algorithm” for anything that goes wrong. But such an explanation tells us nothing about where fault actually lies, and tends to mask a far more complex picture. The blame may indeed lie with the coding, but it is equally likely to lie in the selection of the training dataset, the robustness of the testing prior to deployment, the parameters used (and omitted) during configuration, or the excessive extent to which users of the solution have relied on its outputs.
How does a prospective claimant unpick this complexity? The first challenge is in identifying what exactly it is that they are concerned about. So, for example, in the case of the A-level grading situation, the overall picture was a positive one. A government press release on the morning of the exam results being published trumpeted an increase in the number of A* and A grades having been awarded. It also emphasised that the majority of grades awarded were the same, or within one grade of, centre assessment grades. This, however, masked a more complicated (and negative) picture in the detail of the figures. A large number of individuals were dissatisfied with their grades. In looking to challenge these, they were being told that they could, as an individual, appeal against the grading, in which case their individual position could be reviewed. Or they could sit an exam.
While it is always important for there to be scope to appeal to human oversight against algorithmic decision-making (indeed it is essentially mandatory when personal data is in play; ie GDPR Art 22(1) provides that “The data subject shall have the right not to be subject to a decision based solely on automated processing, including profiling, which produces legal effects concerning him or her or similarly significantly affects him or her”), an individual appeal about the conclusion that the algorithm has reached is highly unlikely to be the vehicle to address any wider concerns about systematic unfairness. This is why, even before the end of the day on which the results were published, efforts were already in train to mobilise a group claim on behalf of the affected students as a whole, looking to challenge the adoption of the system in the first place, and the way in which it had been implemented.
How can redress be sought?
Such a claim can be extremely challenging to mount. First, it is necessary to show that it is the algorithm that has produced the unfairness. So, to take a different example, one of the obstacles for those who sought to challenge the adoption of facial recognition technology, has been to demonstrate evidentially that the systems they object to produce measurably worse outcomes for certain racial, ethnic or gender groups compared to others. This requires first obtaining the raw data outputs from the system in operation, and then obtaining expert evidence to identify in what quantifiable ways individuals or groups have been discriminated against. It has taken most of the last two decades to put together thorough, broad-based research on this issue.
The second challenge is to identify what law has been infringed. This is, perhaps, more straightforward when it comes to outcomes which are worse for a group that are defined by a protected characteristic. Discrimination on the grounds of race is, by definition, unlawful, in a way that discrimination on the basis of demographic circumstances is not always. So, for example, it is not as easy to pinpoint a breach of the law in a system which produced outcomes that varied based on attributes such as poverty/affluence, or state/private education.
Having identified, if they can, what the claimant group are complaining about, they then need to work out how to pursue their complaint. We saw above that challenging the individual-level decision is seldom the right way to go, but for the individual in question, there will be powerful incentives for them to focus their efforts there. Let’s imagine that a group of individuals claiming benefits from their local authority are profiled by an algorithm which is intended to help identify potential fraudsters. If an individual is accused of seeking to defraud the benefits system then, leaving aside the significant disadvantage that such a person might have in sourcing legal advice and representation in the first place, their focus is inevitably going to be on exonerating themselves, rather than tackling the system that implicated them. Even if they were to mount a broader challenge, it would be directed to the local authority that deployed the software, rather than the provider (let alone the developer) of the software itself.
What, in those circumstances, can a local authority do? Whether or not they are on the receiving end of a viable claim for compensation, it can be assumed that no local authority would deliberately have set out wrongly to accuse its citizens of fraud. The authority might therefore look to seek redress under their contract with the provider, but such contracts often promise only nebulous results; generally speaking such software is provided expressly on the basis that it is intended to “support” human decision-making, rather than offering any guarantees about the accuracy of the decisions. For many, it becomes a question of using the imprecise levers of market-forces: cancelling contracts or declining to renew or extend existing programmes. But even if such an approach limits the scope for further harm, it is unlikely to provide any satisfactory redress for those injured in the first place, nor in itself to help us get any closer to a model for holding such systems to account.
That latter challenge is becoming an increasingly urgent priority. The buyers of such tools (be they businesses, public bodies or governments) are not adopting algorithms because they relish the rise of an automated over-class who will determine what is best for its human subjects. Rather they are driven to adopt such systems, often with considerable misgivings, because they are seen to be the only way to deliver the outcomes that they have been tasked with securing, on the limited budgets that they have been left to work with.
In that context, although many of the systems described above will have been sold as “support” tools, the reality is that under-resourced users are going to depend to a significant degree on the guidance that the system produces. That dependency on the system only increases, the more the case for deployment of technology increases. A small/medium business with dozens of personnel probably doesn’t need a sophisticated AI tool to help them to identify opportunities for down-sizing. But organisations with thousands, or tens of thousands of employees may have no other way of identifying the areas of greatest inefficiency. Just as scale can present a challenge, so can time. So one of the first areas in which automated tools rose to prominence (and notoriety) was in the field of financial transactions and in particular commodity and currency trading. Here, margins of a fraction of a second could make the difference between profit and loss. And the marginal gains achieved a small automated advantage on every trade rapidly add up to substantial gains, when taken across a firm’s whole book of business.
The law needs to catch up
At the moment though, the law does not reflect the degree of dependence that businesses, authorities and individuals place on those systems. It is still permissible, indeed commonplace, for providers of AI/algorithmic tools to supply them on contractual terms that are more suited to old-fashioned software. So sophisticated tools, the workings of which buyers are very poorly equipped to understand, let alone evaluate, are sold on terms that regardless of the harm done by the system, the vendor’s liability is capped to a high extent, or excluded as far as permissible by law. And that doesn’t even start to consider the complexities that are introduced when the code in question has been developed, not by a human development team, but by an AI coding tool. When the outputs of such systems can be used to determine questions relevant to someone’s liberty or reputation, or their future employment prospects, or can be fatal to a business’s livelihood, the availability of meaningful redress is important. Not just so that those specific individuals or businesses are compensated properly for the harm done to them; but because the existence of a meaningful remedy drives improvement in the way that such tools are developed and sold.
On 1 August 2012, a highly experienced automated trading firm called Knight Capital started testing a new piece of algorithmic software on the New York Stock Exchange. Despite precautions, it started executing loss-making trades, at a rate of nearly 2,500 times a minute. At the end of a 45 minute period it had lost nearly $440m dollars. Within months, it had ended up being bought up by a competitor. As a reporter at the time put it, “I think we’ll find that the culprit was a combination of ISV software bugs, bad documentation, and human error from Knight Capital. In short, plenty of blame to go around”.
Nearly a decade further on, we have not got any better at pinpointing how blame should be allocated in the failure of such complicated systems, while their complexity (and the stakes if they go wrong) has only continued to increase. What is clear, though, is that the absence of robust liability for the designers of such tools, coupled with pressure on the part of buyers to “move fast and break things” in response to time and resource constraints, is continuing to create circumstances in which such failures will continue. Unless the root causes of that are addressed, through education, through more rigorous testing, through law and regulation, we are likely to hear a lot more talk of “mutant algorithms” being at fault. In reality, however, there will remain plenty of blame to go around among the humans as well.
Will Richmond-Coggan is a director in the data protection team at Freeths LLP. He acts for clients from start-ups to multinationals on a wide range of strategic, commercial and contentious data protection and privacy issues. Email William.Richmond-Coggan@freeths.co.uk. Twitter @Tech_Litig8or.